Palmprints are private and stable information for biometric recognition. In the deep learning era, the development of palmprint recognition is limited by the lack of sufficient training data. In this paper, by observing that palmar creases are the key information to deep-learning-based palmprint recognition, we propose to synthesize training data by manipulating palmar creases. Concretely, we introduce an intuitive geometric model which represents palmar creases with parameterized Bézier curves. By randomly sampling Bézier parameters, we can synthesize massive training samples of diverse identities, which enables us to pretrain large-scale palmprint recognition models. Experimental results demonstrate that such synthetically pretrained models have a very strong generalization ability: they can be efficiently transferred to real datasets, leading to significant performance improvements on palmprint recognition. For example, under the open-set protocol, our method improves the strong ArcFace baseline by more than 10% in terms of TAR@1e-6. And under the closed-set protocol, our method reduces the equal error rate (EER) by an order of magnitude.
BézierPalm: A Free Lunch for Palmprint Recognition
1. Tencent Youtu Lab,
2. UCLA,
3. Hefei University of Technology,
4. Shanghai Jiaotong University.
Abstract:
Highlights:
- We observe that palm creases play an important role in deep learning-based palmprint recognition;
- We propose to synthesize palmar images by manipulating palm creases;
- Bézier curves are used to simulate the palm creases and synthesize samples of diverse identities by controlling the parameters of Bézier curves.
- The synthesized images are used to pre-train palm recognition models, and then finetune on real palm datasets. Experimental results demonstrate that our synthetically pretrained models generalize well on real palm datasets and substantially improve recognition performance.
ECCV2022 slides and presentation:
What's new
- Our paper has been accepted to ECCV2022👏👏.
- We have open-sourced the data synthesizing codes. Checkout it on GitHub.
- We released our paper (pdf).
Synthesized Examples:
Synthesize in 2D:
The figure below provides some synthesized samples, each row contains sample of the same identity.
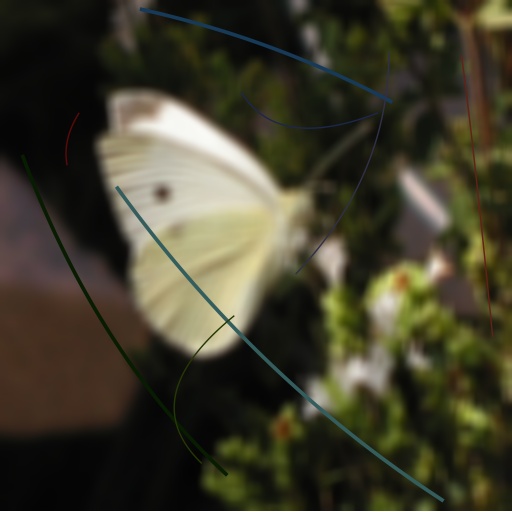
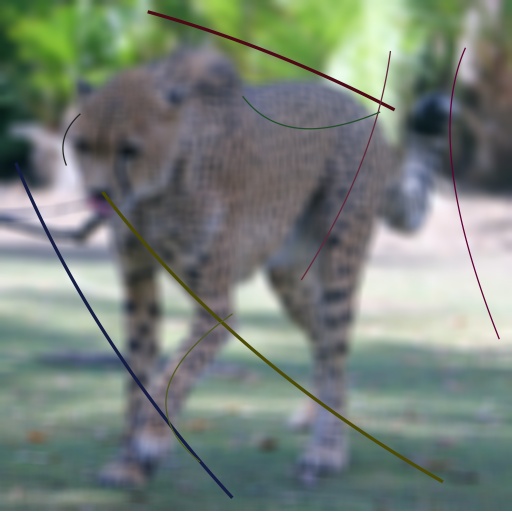
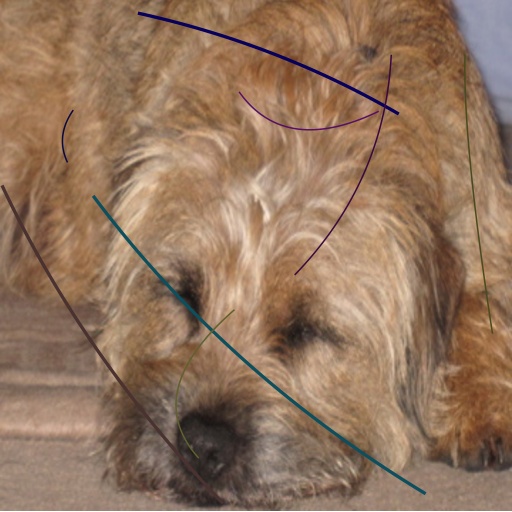
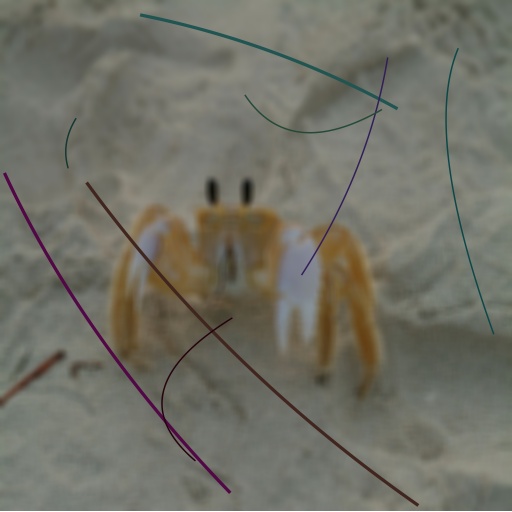
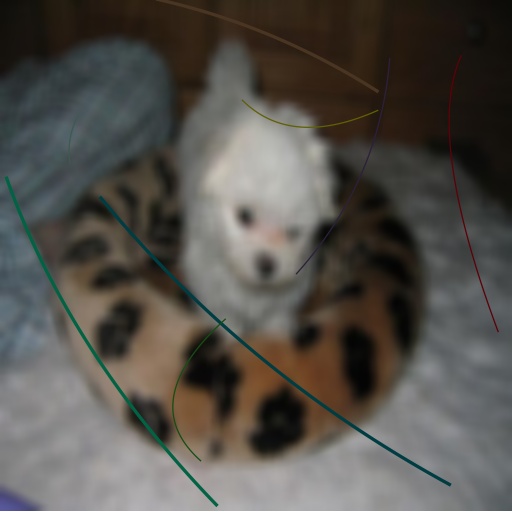
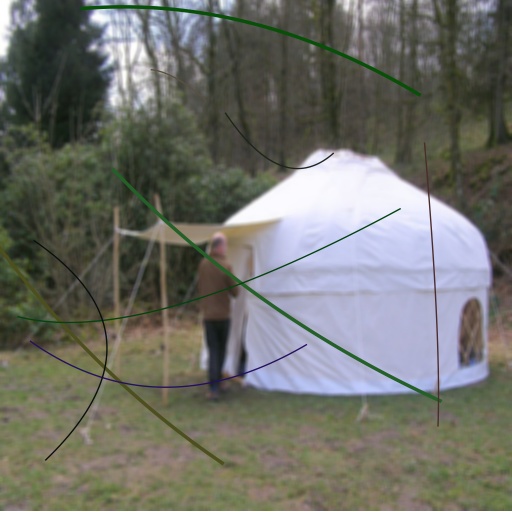
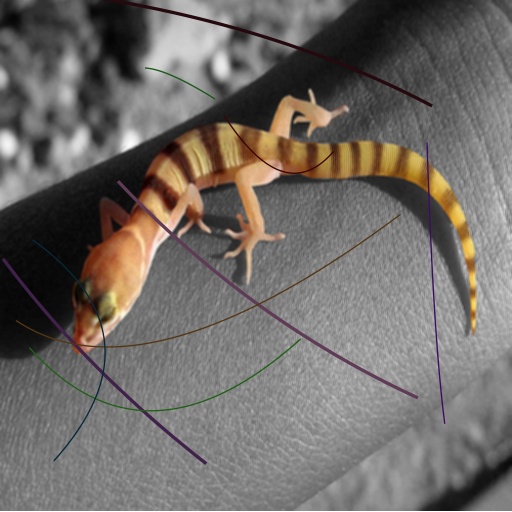
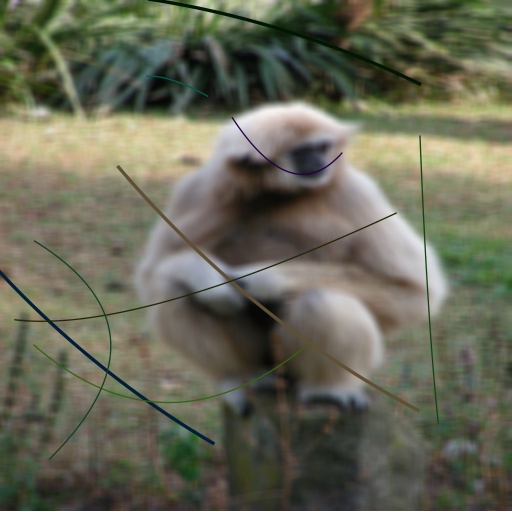
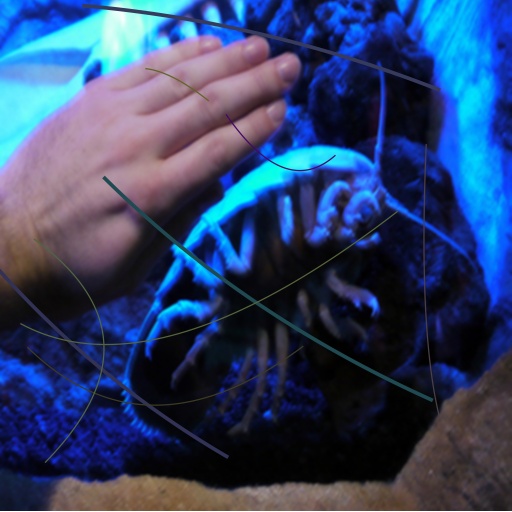
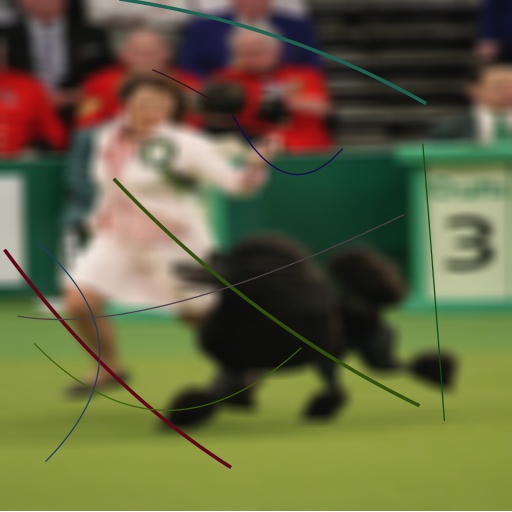
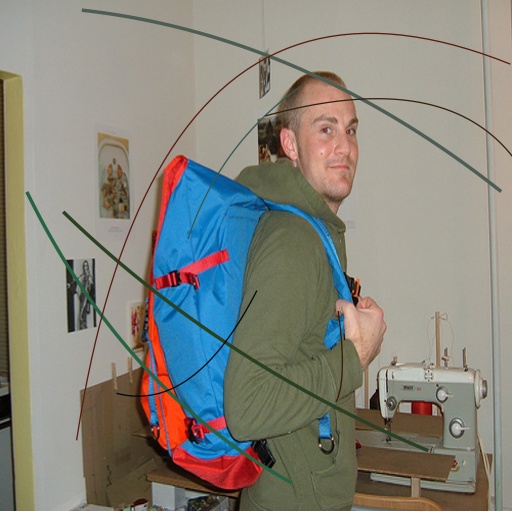
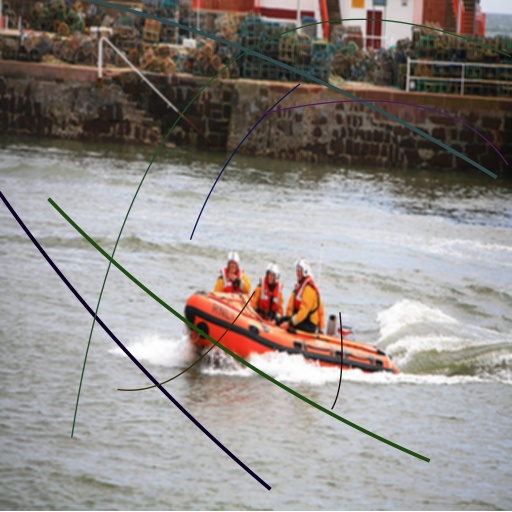
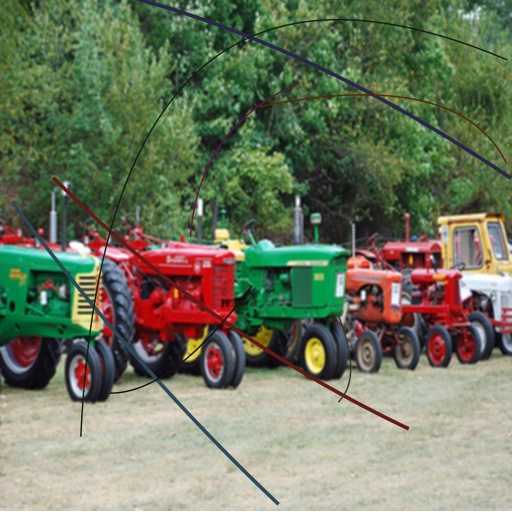
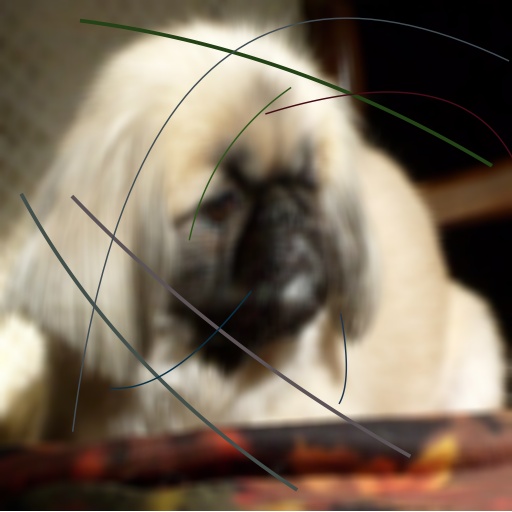
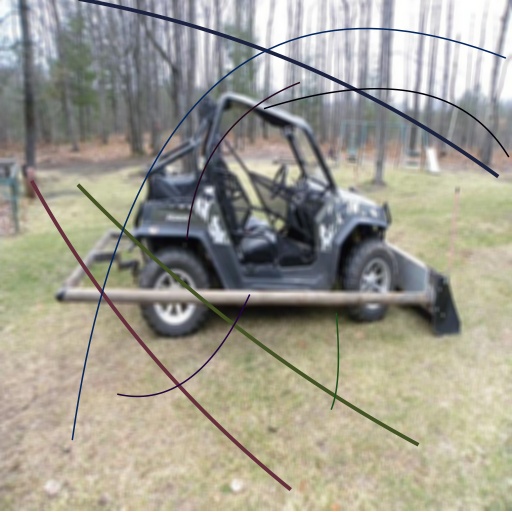
Synthesize in 3D:
Coming soon.
Experimental Results
TAR@FAR curve on public datasets.
Our method consistently outperforms the baseline with substantial margin.
ImageNet pretrained v.s. our synthetically pretrained.
Compared to ImageNet pretraining, our synthetically pretrained models generalize better when finetuning on real palmprint recognition datasets.
Citation:
If our methods are helpful to your research, please kindly consider to cite:@InProceedings{zhao2022bezier,
author = {Zhao, Kai and Shen, Lei and Zhang, Yingyi and Zhou Chuhan and Wang, Tao and Ruixin Zhang and Ding Shouhong and Jia, Wei and Shen, Wei},
title = {B\'{e}zier{P}alm: A Free lunch for Palmprint Recognition},
booktitle = {European Conference on Computer Vision (ECCV)},
month = {Oct},
year = {2022},
}
@article{shen2022distribution,
title={Distribution Alignment for Cross-device Palmprint Recognition},
author={Shen, Lei and Zhang, Yingyi and Zhao, Kai and Zhang, Ruixin and Shen, Wei},
year={2022}
}